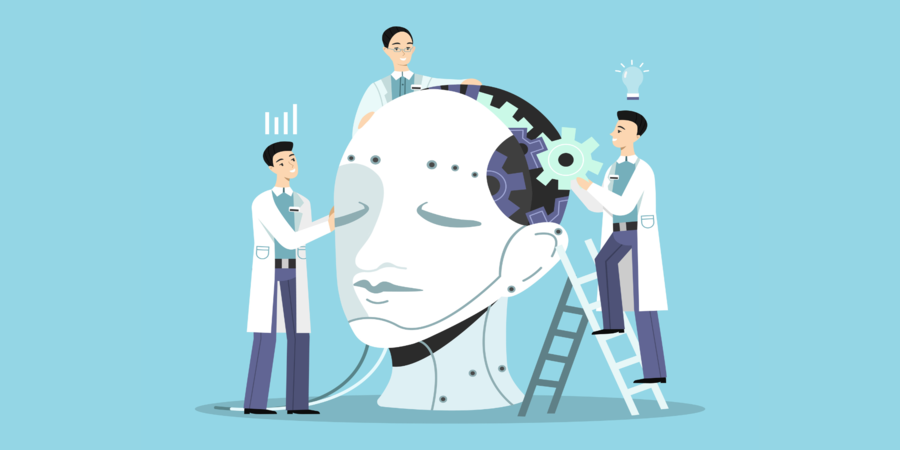
What is Bias in AI?
Artificial Intelligence (AI) has been making incredible strides over the past few years, with its applications being implemented in almost every industry. From chatbots that assist customer service to autonomous cars, AI has revolutionized the way we live our lives. However, as advanced as AI may seem, it still has its flaws. One of the most significant issues in AI is bias. In this article, we will discuss what bias in AI is, how it occurs, and its impact on society.
Defining Bias in AI Bias in AI refers to the systematic and repeatable errors in the AI model's decision-making processes. These errors can result in unfair treatment or disadvantage to certain groups of people based on their race, gender, age, religion, sexual orientation, or other factors. In simpler terms, AI can be biased if it has been trained on data that contains stereotypes or discriminatory patterns.
There are many different kinds of bias in AI, such as:
How Bias Occurs in AI Bias in AI can occur in several ways. The most common way is through the data used to train the AI model. If the data is not diverse enough, or if it contains biases, the AI model will learn those biases and incorporate them into its decision-making process. For example, if an AI model is trained on data that contains primarily male names, it may have difficulty recognizing female names.
Another way bias can occur is through the algorithms used to build the AI model. If the algorithms are not designed to be unbiased, the AI model will make biased decisions. This can happen when the algorithms are not designed with diversity in mind or when they are not tested for fairness.
The Impact of Bias in AI The impact of bias in AI can be significant. It can lead to unfair treatment or disadvantage for certain groups of people. For example, if an AI model is used to evaluate job applications, and it has been trained on data that contains gender biases, it may end up rejecting more female applicants than male applicants.
Bias in AI can also perpetuate existing social inequalities. For example, if an AI model is used to determine loan eligibility, and it has been trained on data that contains racial biases, it may end up approving more loans for white people than people of color.
How to Address Bias in AI Addressing bias in AI requires a multifaceted approach. Here are some steps that can be taken:
Bias in AI is a pressing concern that needs to be addressed to ensure the ethical and fair use of AI technologies. Understanding the different types of bias and how it can occur in AI systems is the first step toward mitigating its impact. By diversifying data, testing for fairness, increasing diversity in development teams, conducting regular audits, establishing ethical frameworks, encouraging user feedback, and promoting collaboration and regulation, we can work towards building AI systems that are unbiased and beneficial for all of society. It is essential that we strive for transparency, accountability, and fairness in AI to harness its true potential while minimizing the risks associated with bias.
Popular articles
Jun 08, 2023 07:51 AM
Jun 08, 2023 08:05 AM
Jun 08, 2023 03:04 AM
Jun 07, 2023 04:32 AM
Jun 05, 2023 06:41 AM
Comments (0)