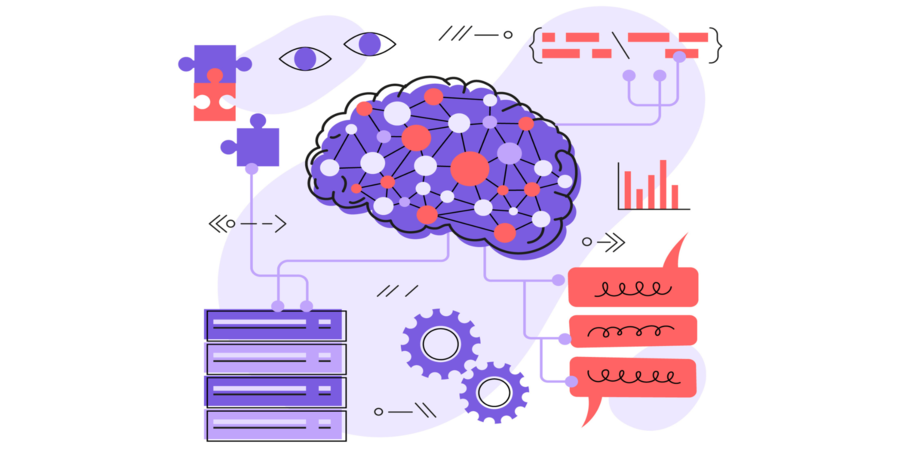
What is deep learning And How Does It Work?
Deep learning has been one of the most transformative technologies of the last decade. This type of artificial intelligence has enabled machines to learn from data, and has powered new applications in fields ranging from medicine to finance. In this article, we will explore the basics of deep learning, including its history, underlying principles, and current applications.
Deep learning is often considered a subset of machine learning, which has its origins in the midtwentieth century. In the late 1950s, a group of researchers began exploring the possibility of training machines to perform simple tasks, such as recognizing hand-written letters. These early efforts were based on a concept called artificial neural networks (ANNs), which mimic the structure of the human brain.
However, despite some early successes, machine learning research was largely stalled for several decades. This was due to a variety of factors, including a lack of funding and computing power.
The breakthrough for deep learning came in the 2000s, when researchers developed new algorithms and hardware that allowed ANNs to become much deeper and more complex. This enabled machines to learn more sophisticated patterns and relationships in data, leading to a surge in interest and investment in deep learning.
Deep learning is a subset of machine learning that involves the use of neural networks to learn and make decisions based on data. It has become a popular approach to solving a wide range of complex problems, from image recognition to natural language processing. In this article, we will explore how deep learning works and the key concepts behind it.
At the heart of deep learning are neural networks. These are mathematical models that are inspired by the structure and function of the human brain. Neural networks are composed of layers of interconnected nodes, each of which performs a specific computation. When data is input into the network, it passes through the layers of nodes, with each layer performing a different type of computation, until it produces an output.
Before a neural network can be used for a specific task, it must be trained on a large dataset. During the training process, the network learns to recognize patterns in the data and make predictions based on those patterns. This is done by adjusting the weights of the connections between nodes in the network to minimize the difference between the predicted output and the actual output.
Once a neural network has been trained, it can be used to make predictions on new data. For example, a deep learning model trained on images of cats and dogs could be used to identify whether a new image contains a cat or a dog. The model would analyze the image, recognize patterns that are indicative of cats or dogs, and make a prediction based on those patterns.
At its core, deep learning is based on a simple idea: instead of writing explicit instructions for a machine, we can train it to learn from examples. This is done by creating a neural network, which is a series of interconnected layers that process input data and output predictions.
A key element of deep learning is backpropagation, which is a method for updating the weights in the neural network. In backpropagation, the network is trained by comparing the output predictions with the true labels and adjusting the weights to minimize the difference between them. This process is repeated many times, until the network achieves a high level of accuracy.
There are several different types of neural networks used in deep learning, including feedforward networks, convolutional networks, and recurrent networks. Each of these has its own strengths and weaknesses, and is used in different applications depending on the nature of the data.
Deep learning has the potential to transform a wide range of industries, and is already being used in applications ranging from self-driving cars to medical diagnosis. Here are just a few examples:
Despite its many successes, deep learning still has a number of challenges to overcome. One of the main challenges is the need for large amounts of labeled data, which can be expensive and time-consuming to collect. Another challenge is the lack of transparency in the decision-making process of deep learning algorithms, which can make it difficult to understand how they are making predictions.
To address these challenges, researchers are exploring new techniques such as transfer learning and unsupervised learning. Transfer learning involves using a pre-trained model to transfer knowledge to a new task, while unsupervised learning involves training neural networks on data without labels.
In the future, deep learning is likely to continue to have a major impact on a wide range of industries. As new applications emerge and technology continues to improve, we can expect to see even more exciting developments in the field of deep learning.
In conclusion, deep learning is a rapidly growing and evolving field of artificial intelligence that is changing the way we interact with machines and the world around us. By enabling machines to learn from data, deep learning is powering new applications and solutions in industries ranging from healthcare to finance.
While deep learning has achieved many successes, it still faces challenges such as the need for large amounts of labeled data and the lack of transparency in decision-making. However, researchers are exploring new techniques to address these challenges and continue to push the boundaries of what deep learning can achieve.
Overall, deep learning is an exciting and important area of research with significant potential to impact society and transform industries. As we continue to develop and refine this technology, we can expect to see even more innovative and creative applications of deep learning in the years to come.
Popular articles
Jun 08, 2023 07:51 AM
Jun 08, 2023 08:05 AM
Jun 08, 2023 03:04 AM
Jun 07, 2023 04:32 AM
Jun 05, 2023 06:41 AM
Comments (0)