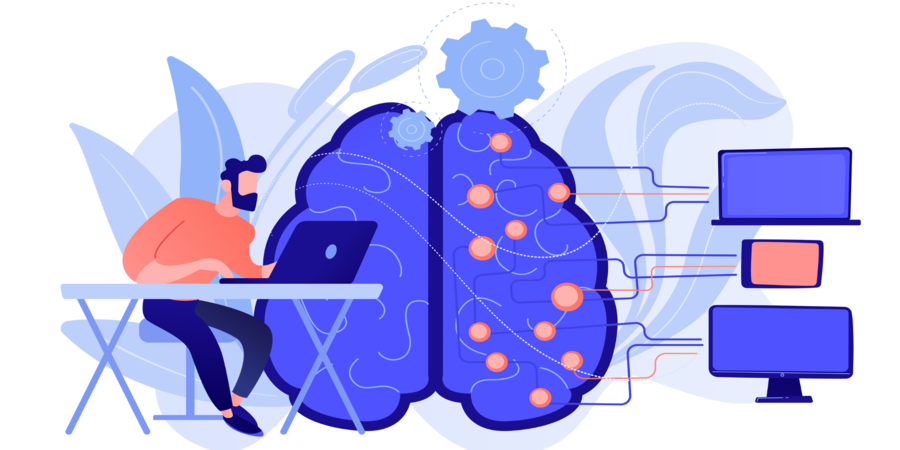
What is Explainable AI (XAI)?
Machine learning models have become increasingly sophisticated, able to make predictions and decisions with high accuracy. However, the more complex and opaque these models become, the harder it is to understand how they arrived at their conclusions. This lack of transparency is particularly problematic when the decisions made by these models have significant consequences, such as in healthcare, finance, and criminal justice. Explainable AI (XAI) is a field of research that seeks to address this issue by making machine learning models more transparent and interpretable.
Explainable AI (XAI) is a collection of approaches that aim to improve the interpretability and openness of machine learning models for human users. These techniques aim to provide insights into how a model makes predictions or decisions, the factors that influence those decisions, and the reasoning behind them.
The goal of XAI is to increase the transparency and accountability of machine learning models, making them more trustworthy and easier to use in real-world applications. By providing a clearer understanding of how a model works, XAI can help to identify and address potential biases, errors, and ethical issues that may arise from its use.
As machine learning models become increasingly complex and opaque, it becomes more difficult for humans to understand how they work. This lack of transparency can lead to a lack of trust in the models, as well as potential ethical concerns. For example, in healthcare, a model that makes decisions about patient care without providing an explanation for those decisions may lead to mistrust from healthcare providers and patients alike.
Similarly, in finance, a model that makes decisions about creditworthiness or loan approvals without providing an explanation may be perceived as biased or unfair. By making machine learning models more transparent and interpretable, XAI can help to address these concerns and increase the trustworthiness of the models.
There are several techniques used in XAI to make machine learning models more transparent and interpretable. These include:
Feature Importance: This technique involves identifying the features or variables in a dataset that are most important in influencing the predictions or decisions made by a model. By highlighting these features, it is possible to gain insights into how the model works and identify potential biases or errors.
Model Visualization: Visualization of models is a method for illustrating how a model makes decisions.. This can include decision trees, flowcharts, or other graphical representations that help to explain how the model arrived at its conclusions.
Local Explanations: This technique involves providing explanations for individual predictions or decisions made by a model. By providing a detailed explanation of how a particular prediction was made, it is possible to increase transparency and identify potential biases or errors.
Simplification: This technique involves simplifying a complex model to make it more interpretable. This can include reducing the number of features or variables used by the model, or using simpler algorithms that are easier to understand.
Increased Trustworthiness: By providing insights into how a model works, XAI can increase trust in the model's predictions or decisions.
Improved Accountability: XAI can help to identify potential biases or errors in a model's decision-making process, making it easier to hold the model accountable for its decisions.
Better Understanding: XAI can help to increase understanding of the factors that influence a model's decision-making process, which can be useful in developing more accurate and effective models.
Increased Complexity: Adding XAI techniques to a model can increase its complexity and reduce its performance. In some cases, the added complexity can make the model less accurate or less efficient.
Trade-offs: There may be trade-offs between explainability and other important factors such as accuracy, performance, and scalability. For example, a model that is highly accurate may be less explainable than a simpler model with lower accuracy.
Lack of Standardization: There is currently no standardized approach to XAI, which can make it difficult to compare and evaluate different techniques. This lack of standardization can also make it challenging to implement XAI in practice.
Explainable AI (XAI) has many potential applications in various industries, including healthcare, finance, and criminal justice.
In healthcare, XAI can help to increase transparency and trust in machine learning models used for diagnosis, treatment, and patient care. By providing explanations for decisions made by these models, XAI can help to identify potential biases and errors, improving the accuracy and reliability of these models.
In finance, XAI can help to increase transparency and accountability in machine learning models used for credit scoring, fraud detection, and investment management. By providing explanations for decisions made by these models, XAI can help to identify potential biases and errors, reducing the risk of unfair or discriminatory practices.
In criminal justice, XAI can help to increase transparency and fairness in machine learning models used for risk assessment, sentencing, and parole decisions. By providing explanations for decisions made by these models, XAI can help to identify potential biases and errors, reducing the risk of unfair or discriminatory practices.
Explainable AI (XAI) is an important field of research that seeks to make machine learning models more transparent and interpretable. By providing insights into how a model works and the reasoning behind its decisions, XAI can help to increase the trustworthiness and accountability of these models. While there are limitations to XAI, including increased complexity and potential trade-offs, the benefits of XAI are significant, and the potential applications in various industries are vast. As machine learning models become increasingly ubiquitous, XAI will continue to play a critical role in ensuring their reliability, fairness, and ethical use.
Popular articles
Jun 08, 2023 07:51 AM
Jun 08, 2023 08:05 AM
Jun 08, 2023 03:04 AM
Jun 07, 2023 04:32 AM
Jun 05, 2023 06:41 AM
Comments (0)